How Large Language Models Are Revolutionizing the Analysis of Public User Social Accounts and Building Communities of Shared Interests
- Karkadan Karkadan
- Jan 31
- 5 min read
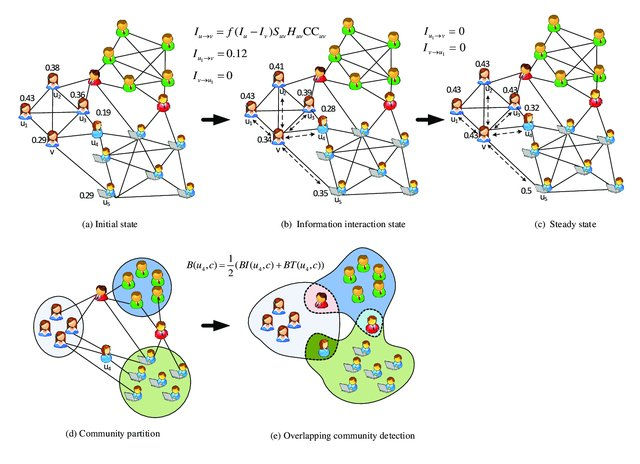
In the age of digital transformation, social media platforms like Instagram, YouTube, LinkedIn, and others have become treasure troves of user-generated content. From images and videos to text posts, hashtags, and even podcasts, these platforms are rich with data that reflects the interests, behaviors, and demographics of millions of users. However, the sheer volume and complexity of this data make it nearly impossible for traditional methods to extract meaningful insights. This is where large language models (LLMs) and transformer-based architectures come into play. These advanced technologies are not only capable of processing multimodal data—such as images, text, and audio—but also of uncovering hidden patterns and building communities of users who share similar interests, even when those interests are deeply embedded in the data.
The Multimodal Challenge of Social Media Data
Social media platforms are inherently multimodal, meaning they generate and host data in various forms. For instance, an Instagram post might include an image, a caption, hashtags, geolocation tags, and even user comments. Similarly, a YouTube video combines visual content, audio, a title, a description, and a comment section. LinkedIn posts often merge text with professional metadata, such as job titles, company affiliations, and shared articles. Podcasts, as a newer and increasingly popular format, add another layer of complexity by delivering rich audio content that can be analyzed for tone, sentiment, and even demographic cues. Each of these modalities provides a unique lens through which user behavior and interests can be understood.
Traditional data analysis methods struggle to handle this complexity. For example, a text-based model might analyze the caption of an Instagram post but fail to account for the visual content of the image or the context provided by hashtags. Similarly, an image recognition model might identify objects in a photo but miss the nuanced meaning conveyed by the accompanying text. This is where large language models, particularly those built on transformer architectures, excel. Unlike traditional models that focus on a single type of data, LLMs can process and integrate multiple modalities simultaneously. For instance, a transformer-based model can analyze an Instagram post by extracting visual features from the image, understanding the semantics of the caption, and interpreting the context of the hashtags. By combining these insights, the model can form a holistic understanding of the post and the user who created it.
Uncovering Hidden Interests and Building Communities
One of the most powerful applications of LLMs in social media analysis is their ability to uncover hidden interests and build communities around shared preferences. Often, user interests are not explicitly stated but are embedded in the content they create and consume. For example, a user might post a picture of a hiking trail without explicitly mentioning their love for outdoor activities. Similarly, a podcast listener might not state their demographic preferences, but their choice of content can reveal insights about their age, gender, or cultural background.
LLMs excel at identifying these hidden patterns. By analyzing the text, images, and metadata associated with user posts, these models can infer interests that are not immediately obvious. For instance, a model might detect that users who post about hiking trails also frequently use hashtags related to environmental conservation or fitness. By connecting these dots, the model can identify a community of users who share a passion for outdoor activities and sustainability. This process is akin to the community detection techniques described by Alfaqeeh and Skillicorn (2023) in their work on spectral embedding of typed graphs, where they emphasize the importance of integrating multiple data types to uncover latent community structures in social networks (Alfaqeeh & Skillicorn, 2023).
This process of community building is further enhanced by the ability of LLMs to analyze large-scale data. Social media platforms host billions of posts, comments, and interactions, making it impossible for humans to manually identify trends. LLMs, however, can process this data at scale, identifying clusters of users with similar interests and behaviors. These clusters can then be used to build targeted communities, whether for marketing purposes, content recommendations, or social networking. For example, a brand might use these insights to create targeted advertising campaigns for users who share a common interest in sustainable living, or a content creator might tailor their posts to resonate with a specific demographic.
The Role of Podcasts in Demographic Analysis
Podcasts represent a relatively new but increasingly important format of social media data. Unlike text or image-based content, podcasts are rich in audio information, which can be analyzed for tone, sentiment, and even demographic cues. For example, the language used by a podcast host, the topics discussed, and the style of delivery can all provide insights into the target audience.
However, analyzing podcasts goes beyond understanding the content itself. Advanced demographic analysis is often more valuable than the podcast content alone. LLMs can process audio data to infer characteristics such as the age, gender, and cultural background of the listeners. For instance, a podcast that frequently discusses retirement planning and uses a formal tone is likely targeting an older audience. Similarly, a podcast with casual language and topics related to pop culture might appeal to a younger demographic.
By combining this demographic analysis with other modalities—such as user comments, subscription data, and social media interactions—LLMs can build a comprehensive profile of podcast listeners. This information can be used to tailor content recommendations, design targeted advertising campaigns, and even create new podcasts that cater to specific audience segments. For example, a podcast network might use these insights to develop a new show aimed at millennials interested in personal finance, leveraging the demographic and interest data extracted by LLMs.
Challenges and Ethical Considerations
While the capabilities of LLMs in processing social media data are impressive, they also raise important ethical considerations. The ability to infer hidden interests and demographics from user-generated content can be a double-edged sword. On one hand, it enables personalized experiences and community building. On the other hand, it raises concerns about privacy and data security.
Users often share content on social media without fully understanding how it might be analyzed and used. The use of LLMs to extract hidden insights can feel invasive, particularly when it involves sensitive information such as political views, health conditions, or personal preferences. As a result, it is crucial for organizations using these technologies to prioritize transparency and user consent.
Additionally, there is the risk of algorithmic bias. LLMs are trained on large datasets, which may contain biases that can influence the model's outputs. For example, a model trained on social media data might inadvertently reinforce stereotypes or exclude certain demographic groups. Addressing these biases requires careful attention to dataset selection, model training, and ongoing evaluation. Techniques such as those proposed by Alfaqeeh and Skillicorn (2023) in their spectral embedding approach can help mitigate these biases by ensuring that multiple data types are integrated in a balanced and representative manner (Alfaqeeh & Skillicorn, 2023).
Conclusion
Large language models and transformer-based architectures are revolutionizing the way we analyze social media data. By processing multimodal content—such as images, text, and audio—these models can uncover hidden interests, build communities of like-minded users, and provide valuable demographic insights. Podcasts, as a newer format, add another layer of complexity and opportunity, enabling advanced analysis of audio content and listener demographics.
However, the power of these technologies comes with significant responsibilities. Ensuring user privacy, addressing algorithmic bias, and maintaining transparency are essential to harnessing the potential of LLMs in a way that benefits both users and organizations. As social media continues to evolve, the role of large language models in understanding and connecting users will only grow, shaping the future of digital communities and content consumption.
Reference:
Alfaqeeh, M., & Skillicorn, D. B. (2023). Community detection in social networks by spectral embedding of typed graphs. *Social Network Analysis and Mining*, *14*(1), 12. https://doi.org/10.1007/s13278-023-01012-9
Comments